Two-dimensional Taylor Green Vortex
This notebook requires MindSpore version >= 2.0.0 to support new APIs including: mindspore.jit, mindspore.jit_class, mindspore.jacrev.
Overview
In fluid dynamics, the Taylor–Green vortex is an unsteady flow of a decaying vortex, which has an exact closed form solution of the incompressible Navier–Stokes equations in Cartesian coordinates.
Physics-informed Neural Networks (PINNs) provides a new method for quickly solving complex fluid problems by using loss functions that approximate governing equations coupled with simple network configurations. In this case, the data-driven characteristic of neural network is used along with PINNs
to solve the 2D taylor green vortex problem
Technology Path
MindSpore Flow solves the problem as follows:
Training Dataset Construction.
Model Construction.
Multi-task Learning for Adaptive Losses
Optimizer.
NavierStokes2D.
Model Training.
Model Evaluation and Visualization.
Import Necessary Package
[1]:
import time
import numpy as np
import sympy
import mindspore
from mindspore import nn, ops, jit, set_seed
from mindspore import numpy as mnp
The following src
pacakage can be downloaded in applications/physics_driven/navier_stokes/taylor_green/src.
[1]:
from mindflow.cell import MultiScaleFCCell
from mindflow.utils import load_yaml_config
from mindflow.pde import NavierStokes, sympy_to_mindspore
from src import create_training_dataset, create_test_dataset, calculate_l2_error, NavierStokes2D
set_seed(123456)
np.random.seed(123456)
The following taylor_green_2D.yaml
can be downloaded in applications/physics_driven/navier_stokes/taylor_green/configs/taylor_green_2D.yaml.
[2]:
mindspore.set_context(mode=mindspore.GRAPH_MODE, device_target="GPU", device_id=0, save_graphs=False)
use_ascend = mindspore.get_context(attr_key='device_target') == "Ascend"
config = load_yaml_config('taylor_green_2D.yaml')
Dataset Construction
Training dataset is imported through function create_train_dataset, contains domain points, initial condition points and boundary condition point. All datasets are sampled by APIs from mindflow.geometry.
Test dataset is imported through function create_test_dataset. In this case, the exact solution used to construct test dataset is given by J Kim, P Moin, Application of a fractional-step method to incompressible Navier-Stokes equations, Journal of Computational Physics, Volume 59, Issue 2, 1985.
This example considers the aylor-Green eddy current sumulation of a square region of size \(2\pi \times 2\pi\) at the \(t \in (0,2)\) time slot. The exact solution to the problem is:
[2]:
# create training dataset
taylor_dataset = create_training_dataset(config)
train_dataset = taylor_dataset.create_dataset(batch_size=config["train_batch_size"],
shuffle=True,
prebatched_data=True,
drop_remainder=True)
# create test dataset
inputs, label = create_test_dataset(config)
Model Construction
This example uses a simple fully-connected network with a depth of 6 layers and the activation function is the tanh
function.
[3]:
coord_min = np.array(config["geometry"]["coord_min"] + [config["geometry"]["time_min"]]).astype(np.float32)
coord_max = np.array(config["geometry"]["coord_max"] + [config["geometry"]["time_max"]]).astype(np.float32)
input_center = list(0.5 * (coord_max + coord_min))
input_scale = list(2.0 / (coord_max - coord_min))
model = MultiScaleFCCell(in_channels=config["model"]["in_channels"],
out_channels=config["model"]["out_channels"],
layers=config["model"]["layers"],
neurons=config["model"]["neurons"],
residual=config["model"]["residual"],
act='tanh',
num_scales=1,
input_scale=input_scale,
input_center=input_center)
Optimizer
[4]:
params = model.trainable_params()
optimizer = nn.Adam(params, learning_rate=config["optimizer"]["initial_lr"])
Model Training
With MindSpore version >= 2.0.0, we can use the functional programming for training neural networks.
[6]:
def train():
problem = NavierStokes2D(model, re=config["Re"])
if use_ascend:
from mindspore.amp import DynamicLossScaler, auto_mixed_precision, all_finite
loss_scaler = DynamicLossScaler(1024, 2, 100)
auto_mixed_precision(model, 'O3')
def forward_fn(pde_data, ic_data, bc_data):
loss = problem.get_loss(pde_data, ic_data, bc_data)
if use_ascend:
loss = loss_scaler.scale(loss)
return loss
grad_fn = mindspore.value_and_grad(forward_fn, None, optimizer.parameters, has_aux=False)
@jit
def train_step(pde_data, ic_data, bc_data):
loss, grads = grad_fn(pde_data, ic_data, bc_data)
if use_ascend:
loss = loss_scaler.unscale(loss)
if all_finite(grads):
grads = loss_scaler.unscale(grads)
loss = ops.depend(loss, optimizer(grads))
else:
loss = ops.depend(loss, optimizer(grads))
return loss
epochs = config["train_epochs"]
steps_per_epochs = train_dataset.get_dataset_size()
sink_process = mindspore.data_sink(train_step, train_dataset, sink_size=1)
for epoch in range(1, 1 + epochs):
# train
time_beg = time.time()
model.set_train(True)
for _ in range(steps_per_epochs):
step_train_loss = sink_process()
model.set_train(False)
if epoch % config["eval_interval_epochs"] == 0:
print(f"epoch: {epoch} train loss: {step_train_loss} epoch time: {(time.time() - time_beg) * 1000 :.3f} ms")
calculate_l2_error(model, inputs, label, config)
[7]:
start_time = time.time()
train()
print("End-to-End total time: {} s".format(time.time() - start_time))
momentum_x: u(x, y, t)*Derivative(u(x, y, t), x) + v(x, y, t)*Derivative(u(x, y, t), y) + Derivative(p(x, y, t), x) + Derivative(u(x, y, t), t) - 1.0*Derivative(u(x, y, t), (x, 2)) - 1.0*Derivative(u(x, y, t), (y, 2))
Item numbers of current derivative formula nodes: 6
momentum_y: u(x, y, t)*Derivative(v(x, y, t), x) + v(x, y, t)*Derivative(v(x, y, t), y) + Derivative(p(x, y, t), y) + Derivative(v(x, y, t), t) - 1.0*Derivative(v(x, y, t), (x, 2)) - 1.0*Derivative(v(x, y, t), (y, 2))
Item numbers of current derivative formula nodes: 6
continuty: Derivative(u(x, y, t), x) + Derivative(v(x, y, t), y)
Item numbers of current derivative formula nodes: 2
ic_u: u(x, y, t) + sin(y)*cos(x)
Item numbers of current derivative formula nodes: 2
ic_v: v(x, y, t) - sin(x)*cos(y)
Item numbers of current derivative formula nodes: 2
ic_p: p(x, y, t) + 0.25*cos(2*x) + 0.25*cos(2*y)
Item numbers of current derivative formula nodes: 3
bc_u: u(x, y, t) + exp(-2*t)*sin(y)*cos(x)
Item numbers of current derivative formula nodes: 2
bc_v: v(x, y, t) - exp(-2*t)*sin(x)*cos(y)
Item numbers of current derivative formula nodes: 2
bc_p: p(x, y, t) + 0.25*exp(-4*t)*cos(2*x) + 0.25*exp(-4*t)*cos(2*y)
Item numbers of current derivative formula nodes: 3
epoch: 20 train loss: 0.11818831 epoch time: 9838.472 ms
predict total time: 342.714786529541 ms
l2_error, U: 0.7095809547153462 , V: 0.7081305150496081 , P: 1.004580707024092 , Total: 0.7376210740866216
==================================================================================================
epoch: 40 train loss: 0.025397364 epoch time: 9853.950 ms
predict total time: 67.26336479187012 ms
l2_error, U: 0.09177234501446464 , V: 0.14504987645942635 , P: 1.0217915750380309 , Total: 0.3150453016208772
==================================================================================================
epoch: 60 train loss: 0.0049396083 epoch time: 10158.307 ms
predict total time: 121.54984474182129 ms
l2_error, U: 0.08648064925211238 , V: 0.07875554509736878 , P: 0.711385847511365 , Total: 0.2187113170206073
==================================================================================================
epoch: 80 train loss: 0.0018874758 epoch time: 10349.795 ms
predict total time: 85.42561531066895 ms
l2_error, U: 0.08687053366212526 , V: 0.10624717784645109 , P: 0.3269822261697911 , Total: 0.1319986181134018
==================================================================================================
......
epoch: 460 train loss: 0.00015093417 epoch time: 9928.474 ms
predict total time: 81.79974555969238 ms
l2_error, U: 0.033782269766829076 , V: 0.025816595720090357 , P: 0.08782072926563861 , Total: 0.03824859644715835
==================================================================================================
epoch: 480 train loss: 6.400551e-05 epoch time: 9956.549 ms
predict total time: 104.77519035339355 ms
l2_error, U: 0.02242134127961232 , V: 0.021098481157660533 , P: 0.06210985820202502 , Total: 0.027418651376509482
==================================================================================================
epoch: 500 train loss: 8.7400025e-05 epoch time: 10215.720 ms
predict total time: 77.20041275024414 ms
l2_error, U: 0.021138056243295636 , V: 0.013343674071961624 , P: 0.045241559122240635 , Total: 0.02132725837819097
==================================================================================================
End-to-End total time: 5011.718255519867 s
Model Evaluation and Visualization
[5]:
from src import visual
# visualization
visual(model=model, epoch=config["train_epochs"], input_data=inputs, label=label)
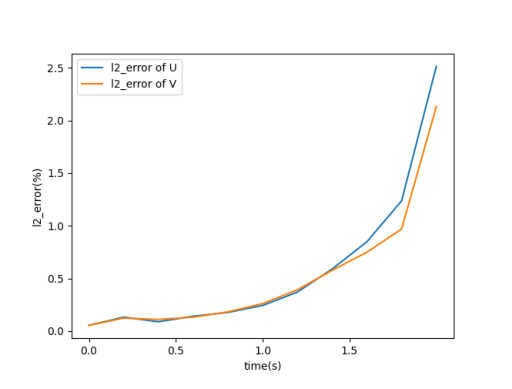
As the speed tends to decrease exponentially, the error becomes larger with time, but the overall is within the 5% error range.