mindspore.dataset
This module provides APIs to load and process various common datasets such as MNIST, CIFAR-10, CIFAR-100, VOC, COCO, ImageNet, CelebA, CLUE, etc. It also supports datasets in standard format, including MindRecord, TFRecord, Manifest, etc. Users can also define their own datasets with this module.
Besides, this module provides APIs to sample data while loading.
We can enable cache in most of the dataset with its key arguments ‘cache’. Please notice that cache is not supported on Windows platform yet. Do not use it while loading and processing data on Windows. More introductions and limitations can refer Single-Node Tensor Cache.
Common imported modules in corresponding API examples are as follows:
import mindspore.dataset as ds
import mindspore.dataset.transforms as transforms
import mindspore.dataset.vision as vision
Descriptions of common dataset terms are as follows:
Dataset, the base class of all the datasets. It provides data processing methods to help preprocess the data.
SourceDataset, an abstract class to represent the source of dataset pipeline which produces data from data sources such as files and databases.
MappableDataset, an abstract class to represent a source dataset which supports for random access.
Iterator, the base class of dataset iterator for enumerating elements.
Introduction to data processing pipeline
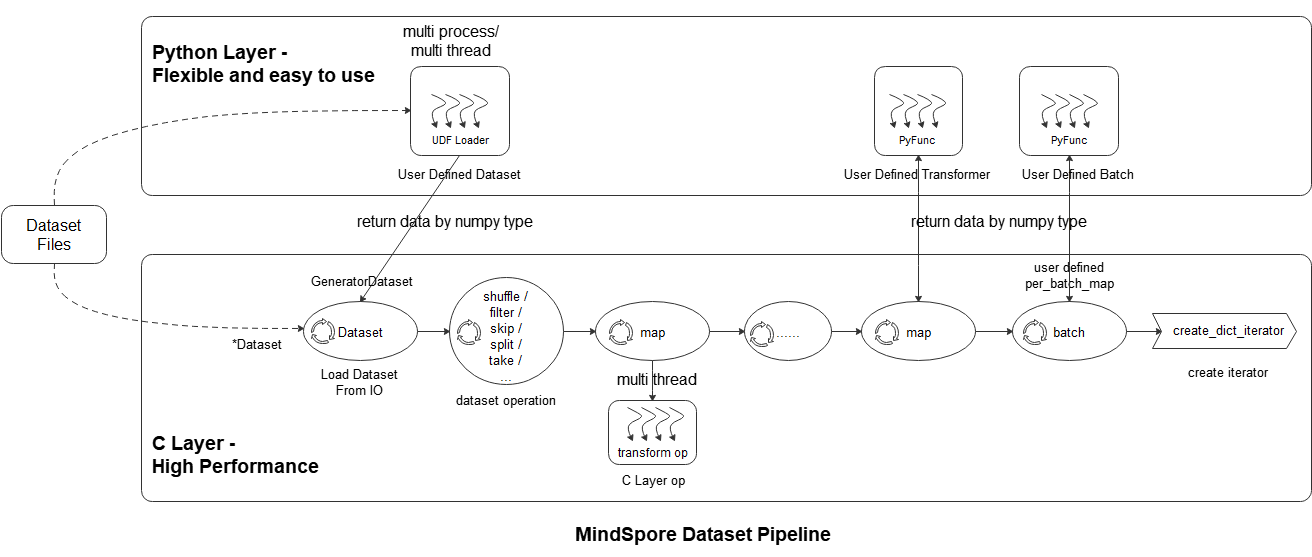
As shown in the above figure, the mindspore dataset module makes it easy for users to define data preprocessing pipelines and transform samples in the dataset in the most efficient (multi-process / multi-thread) manner. The specific steps are as follows:
Loading datasets: Users can easily load supported datasets using the *Dataset class, or load Python layer customized datasets through UDF Loader + GeneratorDataset. At the same time, the loading class method can accept a variety of parameters such as sampler, data slicing, and data shuffle;
Dataset operation: The user uses the dataset object method .shuffle / .filter / .skip / .split / .take / … to further shuffle, filter, skip, and obtain the maximum number of samples of datasets;
Dataset sample transform operation: The user can add data transform operators (vision transform, NLP transform, audio transform) to the map operation to perform transformations. During data preprocessing, multiple map operations can be defined to perform different transform operations to different fields. The data transform operator can also be a user-defined transform pyfunc (Python function);
Batch: After the transformation of the samples, the user can use the batch operation to organize multiple samples into batches, or use self-defined batch logic with the parameter per_batch_map applied;
Iterator: Finally, the user can use the dataset object method create_dict_iterator to create an iterator, which can output the preprocessed data cyclically.
The data processing pipeline example is as follows. Please refer to datasets_example.py for complete example.
import numpy as np
import mindspore as ms
import mindspore.dataset as ds
import mindspore.dataset.vision as vision
import mindspore.dataset.transforms as transforms
# construct data and label
data1 = np.array(np.random.sample(size=(300, 300, 3)) * 255, dtype=np.uint8)
data2 = np.array(np.random.sample(size=(300, 300, 3)) * 255, dtype=np.uint8)
data3 = np.array(np.random.sample(size=(300, 300, 3)) * 255, dtype=np.uint8)
data4 = np.array(np.random.sample(size=(300, 300, 3)) * 255, dtype=np.uint8)
label = [1, 2, 3, 4]
# load the data and label by NumpySlicesDataset
dataset = ds.NumpySlicesDataset(([data1, data2, data3, data4], label), ["data", "label"])
# apply the transform to data
dataset = dataset.map(operations=vision.RandomCrop(size=(250, 250)), input_columns="data")
dataset = dataset.map(operations=vision.Resize(size=(224, 224)), input_columns="data")
dataset = dataset.map(operations=vision.Normalize(mean=[0.485 * 255, 0.456 * 255, 0.406 * 255],
std=[0.229 * 255, 0.224 * 255, 0.225 * 255]),
input_columns="data")
dataset = dataset.map(operations=vision.HWC2CHW(), input_columns="data")
# apply the transform to label
dataset = dataset.map(operations=transforms.TypeCast(ms.int32), input_columns="label")
# batch
dataset = dataset.batch(batch_size=2)
# create iterator
epochs = 2
ds_iter = dataset.create_dict_iterator(output_numpy=True, num_epochs=epochs)
for _ in range(epochs):
for item in ds_iter:
print("item: {}".format(item), flush=True)
Vision
A source dataset that reads and parses Caltech101 dataset. |
|
A source dataset that reads and parses Caltech256 dataset. |
|
A source dataset that reads and parses CelebA dataset. |
|
A source dataset that reads and parses Cifar10 dataset. |
|
A source dataset that reads and parses Cifar100 dataset. |
|
A source dataset that reads and parses Cityscapes dataset. |
|
A source dataset that reads and parses COCO dataset. |
|
A source dataset that reads and parses DIV2KDataset dataset. |
|
A source dataset that reads and parses the EMNIST dataset. |
|
A source dataset for generating fake images. |
|
A source dataset that reads and parses the FASHION-MNIST dataset. |
|
A source dataset that reads and parses Flickr8k and Flickr30k dataset. |
|
A source dataset that reads and parses Flowers102 dataset. |
|
A source dataset that reads images from a tree of directories. |
|
A source dataset that reads and parses the KMNIST dataset. |
|
A source dataset for reading images from a Manifest file. |
|
A source dataset that reads and parses the MNIST dataset. |
|
A source dataset that reads and parses the PhotoTour dataset. |
|
A source dataset that reads and parses the Places365 dataset. |
|
A source dataset that reads and parses the QMNIST dataset. |
|
A source dataset that reads and parses Semantic Boundaries Dataset. |
|
A source dataset that reads and parses the SBU dataset. |
|
A source dataset that reads and parses Semeion dataset. |
|
A source dataset that reads and parses STL10 dataset. |
|
A source dataset that reads and parses SVHN dataset. |
|
A source dataset that reads and parses the USPS dataset. |
|
A source dataset that reads and parses VOC dataset. |
|
A source dataset that reads and parses WIDERFace dataset. |
Text
A source dataset that reads and parses AG News datasets. |
|
A source dataset that reads and parses Amazon Review Polarity and Amazon Review Full datasets. |
|
A source dataset that reads and parses CLUE datasets. |
|
A source dataset that reads and parses CoNLL2000 chunking dataset. |
|
A source dataset that reads and parses comma-separated values (CSV) files as dataset. |
|
A source dataset that reads and parses the DBpedia dataset. |
|
A source dataset that reads and parses EnWik9 dataset. |
|
A source dataset that reads and parses Internet Movie Database (IMDb). |
|
A source dataset that reads and parses IWSLT2016 datasets. |
|
A source dataset that reads and parses IWSLT2017 datasets. |
|
A source dataset that reads and parses PennTreebank datasets. |
|
A source dataset that reads and parses Sogou News dataset. |
|
A source dataset that reads and parses datasets stored on disk in text format. |
|
A source dataset that reads and parses UDPOS dataset. |
|
A source dataset that reads and parses WikiText2 and WikiText103 datasets. |
|
A source dataset that reads and parses the YahooAnswers dataset. |
|
A source dataset that reads and parses Yelp Review Polarity and Yelp Review Full dataset. |
Audio
A source dataset that reads and parses LJSpeech dataset. |
|
A source dataset that reads and parses the SpeechCommands dataset. |
|
A source dataset that reads and parses Tedlium dataset. |
|
A source dataset that reads and parses the YesNo dataset. |
Standard Format
A source dataset that reads and parses comma-separated values (CSV) files as dataset. |
|
A source dataset that reads and parses MindRecord dataset. |
|
A source dataset that reads and parses MindRecord dataset which stored in cloud storage such as OBS, Minio or AWS S3. |
|
A source dataset that reads and parses datasets stored on disk in TFData format. |
User Defined
A source dataset that generates data from Python by invoking Python data source each epoch. |
|
Creates a dataset with given data slices, mainly for loading Python data into dataset. |
|
Creates a dataset with filler data provided by user. |
|
A source dataset that generates random data. |
Graph
Load argoverse dataset and create graph. |
|
A graph object for storing Graph structure and feature data, and provide capabilities such as graph sampling. |
|
Reads the graph dataset used for GNN training from the shared file and database. |
|
Basic Dataset for loading graph into memory. |
Sampler
A sampler that accesses a shard of the dataset, it helps divide dataset into multi-subset for distributed training. |
|
Samples K elements for each P class in the dataset. |
|
Samples the elements randomly. |
|
Samples the dataset elements sequentially that is equivalent to not using a sampler. |
|
Samples the elements randomly from a sequence of indices. |
|
Samples the elements from a sequence of indices. |
|
Samples the elements from [0, len(weights) - 1] randomly with the given weights (probabilities). |
Others
Only the batch size function and per_batch_map of the batch operator can dynamically adjust parameters based on the number of batches and epochs during training. |
|
A client to interface with tensor caching service. |
|
Abstract base class used to build dataset callback classes. |
|
Specifies the sampling strategy when execute get_sampled_neighbors. |
|
Class to represent a schema of a dataset. |
|
Specify the shuffle mode. |
|
Abstract base class used to build dataset callback classes that are synchronized with the training callback class mindspore.Callback . |
|
Specifies the output storage format when execute get_all_neighbors. |
|
Compare if two dataset pipelines are the same. |
|
Construct dataset pipeline from a JSON file produced by dataset serialize function. |
|
Serialize dataset pipeline into a JSON file. |
|
Write the dataset pipeline graph to logger.info file. |
|
Wait util the dataset files required by all devices are downloaded. |
|
Draw an image with given bboxes and class labels (with scores). |
|
Zip the datasets in the input tuple of datasets. |