Performance Profiling (Ascend)
Overview
This article describes how to use MindSpore Profiler for performance debugging on Ascend AI processors.
Operation Process
Prepare a training script, add profiler APIs in the training script and run the training script.
Start MindSpore Insight and specify the summary-base-dir using startup parameters, note that summary-base-dir is the parent directory of the directory created by Profiler. For example, the directory created by Profiler is
/home/user/code/data/
, the summary-base-dir should be/home/user/code
. After MindSpore Insight is started, access the visualization page based on the IP address and port number. The default access IP address ishttp://127.0.0.1:8080
.Find the training in the list, click the performance profiling link and view the data on the web page.
Preparing the Training Script
There are two ways to collect neural network performance data. You can enable Profiler in either of the following ways.
Method 1: Modify the training script
Add the MindSpore Profiler interface to the training script.
Before training, initialize the MindSpore Profiler object, and profiler enables collection of performance data.
Note
The parameters of Profiler are as follows: Profiler API . Before initializing the Profiler, you need to determine the device_id.
At the end of the training,
Profiler.analyse()
should be called to finish profiling and generate the performance analyse results.
** Conditional open example: **
The user decides not to start the Profiler by setting the initialization parameter start_profile to False, then starts the Profiler at the right time by calling Start, stops collecting data, and finally calls analyse to phase the data. You can open and close the Profiler based on the epoch or step, and the data within the specified step or epoch interval is collected. There are two ways to collect performance data based on step or epoch, one is through user-defined training, the other is through Callback based on step or epoch to open and close Profiler.
Custom training:
The MindSpore functional programming use case uses profilers for custom training by turning Profiler performance data on or off during a specified step interval or epoch interval. Enable Profiler's complete code sample based on step.
profiler = ms.Profiler(start_profile=False) data_loader = ds.create_dict_iterator() for i, data in enumerate(data_loader): train() if i==100: profiler.start() if i==200: profiler.stop() profiler.analyse()
User-defined Callback
For data non-sink mode, there is only an opportunity to turn on and off CANN at the end of each step, so whether the CANN is turned on or off is based on the step. A custom Callback opens the Profiler's complete code sample based on step .
import os import mindspore as ms from mindspore.communication import get_rank def get_real_rank(): """get rank id""" try: return get_rank() except RuntimeError: return int(os.getenv("RANK_ID", "0")) class StopAtStep(ms.Callback): def __init__(self, start_step, stop_step): super(StopAtStep, self).__init__() self.start_step = start_step self.stop_step = stop_step # Set the path for performance data to disks as rank_id rank_id = get_real_rank() output_path = os.path.join("profiler_data", f"rank_{rank_id}") self.profiler = ms.Profiler(start_profile=False, output_path=output_path) def on_train_step_begin(self, run_context): cb_params = run_context.original_args() step_num = cb_params.cur_step_num if step_num == self.start_step: self.profiler.start() def on_train_step_end(self, run_context): cb_params = run_context.original_args() step_num = cb_params.cur_step_num if step_num == self.stop_step: self.profiler.stop() self.profiler.analyse()
For data sink mode, CANN is told to start and stop only after the end of each epoch, so it needs to start and stop based on the epoch. The Profiler sample code modification training script can be opened based on step according to a custom Callback.
class StopAtEpoch(ms.Callback): def __init__(self, start_epoch, stop_epoch): super(StopAtEpoch, self).__init__() self.start_epoch = start_epoch self.stop_epoch = stop_epoch # Set the path for performance data to disks as rank_id rank_id = get_real_rank() output_path = os.path.join("profiler_data", f"rank_{rank_id}") self.profiler = ms.Profiler(start_profile=False, output_path=output_path) def on_train_epoch_begin(self, run_context): cb_params = run_context.original_args() epoch_num = cb_params.cur_epoch_num if epoch_num == self.start_epoch: self.profiler.start() def on_train_epoch_end(self, run_context): cb_params = run_context.original_args() epoch_num = cb_params.cur_epoch_num if epoch_num == self.stop_epoch: self.profiler.stop() self.profiler.analyse()
** Unconditional Open example: **
Example 1: In the MindSpore functional Programming use case, Profiler is used to collect performance data. Part of the sample code is shown below. Complete code sample .
# Init Profiler. # Note that the Profiler should be initialized before model training. profiler = Profiler(output_path="profiler_data") def forward_fn(data, label): logits = model(data) loss = loss_fn(logits, label) return loss, logits # Get gradient function grad_fn = ms.value_and_grad(forward_fn, None, optimizer.parameters, has_aux=True) @ms.jit def train_step(data, label): """Define function of one-step training""" (loss, _), grads = grad_fn(data, label) optimizer(grads) return loss for t in range(epochs): train_loop(model, train_dataset, loss_fn, optimizer) profiler.analyse()
Example 2: model.train is used for network training. The complete code is as follows:
import numpy as np from mindspore import nn from mindspore.train import Model import mindspore as ms import mindspore.dataset as ds class Net(nn.Cell): def __init__(self): super(Net, self).__init__() self.fc = nn.Dense(2, 2) def construct(self, x): return self.fc(x) def generator(): for i in range(2): yield (np.ones([2, 2]).astype(np.float32), np.ones([2]).astype(np.int32)) def train(net): optimizer = nn.Momentum(net.trainable_params(), 1, 0.9) loss = nn.SoftmaxCrossEntropyWithLogits(sparse=True) data = ds.GeneratorDataset(generator, ["data", "label"]) model = Model(net, loss, optimizer) model.train(1, data) if __name__ == '__main__': ms.set_context(mode=ms.GRAPH_MODE, device_target="Ascend") # Init Profiler # Note that the Profiler should be initialized before model.train profiler = ms.Profiler(output_path='./profiler_data') # Train Model net = Net() train(net) # Profiler end profiler.analyse()
Method 2: Enable environment variables
Before running the network script, configure Profiler configuration items.
Note:
To enable using environment variables, please set the device ID through the environment variables before the script starts executing. Prohibit using the set_context function to set the device ID in the script.
export MS_PROFILER_OPTIONS='{"start": true, "output_path": "/XXX", "profile_memory": false, "profile_communication": false, "aicore_metrics": 0, "l2_cache": false}'
start (bool, mandatory) - Set to true to enable Profiler. Set false to disable performance data collection. Default value: false.
output_path (str, optional) - Represents the path (absolute path) of the output data. Default value: "./data".
op_time (bool, optional) - Indicates whether to collect operators performance data. Default values: true.
profile_memory (bool, optional) - Tensor data will be collected. This data is collected when the value is true. When using this parameter, op_time must be set to true. Default value: false.
profile_communication (bool, optional) - Indicates whether to collect communication performance data in multi-device training. This data is collected when the value is true. In single-device training, this parameter is not set correctly. When using this parameter, op_time must be set to true. Default value: false.
aicore_metrics (int, optional) - Set the indicator type of AI Core. When using this parameter, op_time must be set to true. Default value: 0.
l2_cache (bool, optional) - Set whether to collect l2 cache data. Default value: false.
timeline_limit (int, optional) - Set the maximum storage size of the timeline file (unit M). When using this parameter, op_time must be set to true. Default value: 500.
data_process (bool, optional) - Indicates whether to collect data to prepare performance data. Default value: false.
parallel_strategy (bool, optional) - Indicates whether to collect parallel policy performance data. Default value: false.
profile_framework (str, optional) - Whether to collect host time, it must be one of ["all", "time", null]. Default: null.
with_stack (bool, optional) - Whether to collect frame host call stack data on the Python side. This data is presented in the form of a flame graph in the timeline. When using this parameter, the parameter op_time must be set to true. Default value: false .
Method 3:Enable Dynamic Profile
mindspore.profiler.DynamicProfilerMonitor provides the ability to dynamically modify the configuration parameters of the profiler without interrupting the training process. The following is an example of the generated JSON configuration file for initialization.
{
"start_step": -1,
"stop_step": -1,
"aicore_metrics": -1,
"profiler_level": -1,
"profile_framework": -1,
"analyse_mode": -1,
"profile_communication": false,
"parallel_strategy": false,
"with_stack": false,
"data_simplification": true
}
start_step (int, mandatory) - Sets the number of steps at which the Profiler will start acquisition, as a relative value, with the first step of training being 1. The default value of -1 means that acquisition will not start throughout the training process.
stop_step (int, mandatory) - Sets the number of steps at which the Profiler starts and stops, as a relative value. The first step of training is 1, which needs to be satisfied that stop_step is greater than or equal to start_step. The default value of -1 means that acquisition will not be started throughout the training process.
aicore_metrics (int, optional) - Set to collect AI Core metrics data, the value range is consistent with Profiler. The default value of -1 means no AI Core metrics are collected.
profiler_level (int, optional) - Sets the performance data collection level, 0 for ProfilerLevel.Level0, 1 for ProfilerLevel.Level1, 2 for ProfilerLevel.Level2. The default value is -1, indicating that the performance data collection level is not controlled.
profile_framework (int, optional) - Set the category of host information to be collected, 0 for “all”, 1 for “time”. Default value is -1, which means no host information will be collected.
analyse_mode (int, optional) - Set the mode of online parsing, it corresponds to the analyse_mode parameter of mindspore.Profiler.analyse interface, 0 means “sync”, 1 means “async”. The default value is -1, which means online parsing is not used.
profile_communication (bool, optional) - Set whether to collect communication performance data in multi-device training. True indicates that communication performance data is collected, while false, the default value, indicates that communication performance data is not collected.
parallel_strategy (bool, optional) - Set whether to collect parallel strategy performance data. True indicates that parallel strategy performance data is collected, while false, the default value, indicates that parallel strategy performance data is not collected.
with_stack (bool, optional) - Set whether to capture call stack information. True indicates that the call stack information is collected, and false, the default value, indicates that the call stack information is not collected.
data_simplification (bool, optional) - Set to enable data simplification, true means on, false means off. The default value is true, which means data simplification is enabled.
Example 1: Use model.train for network training, register DynamicProfilerMonitor to model.train.
Step 1: Add DynamicProfilerMonitor to the training code to register it to the training flow.
import numpy as np from mindspore import nn from mindspore.train import Model import mindspore as ms import mindspore.dataset as ds from mindspore.profiler import DynamicProfilerMonitor class Net(nn.Cell): def __init__(self): super(Net, self).__init__() self.fc = nn.Dense(2, 2) def construct(self, x): return self.fc(x) def generator(): for i in range(2): yield (np.ones([2, 2]).astype(np.float32), np.ones([2]).astype(np.int32)) def train(net): optimizer = nn.Momentum(net.trainable_params(), 1, 0.9) loss = nn.SoftmaxCrossEntropyWithLogits(sparse=True) data = ds.GeneratorDataset(generator, ["data", "label"]) # The cfg_path parameter is the path to the folder where the configuration file is to be shared, which needs to be accessible by all nodes in a cluster scenario # The output_path parameter is the path where the dynamic profile data is saved. profile_callback = DynamicProfilerMonitor(cfg_path="./dyn_cfg", output_path="./dynprof_data") model = Model(net, loss, optimizer) model.train(10, data, callbacks=[profile_callback]) if __name__ == '__main__': ms.set_context(mode=ms.GRAPH_MODE, device_target="Ascend") # Train Mode net = Net() train(net)
Step 2: Pull up the training process and dynamically modify the configuration file to realize dynamic collection of performance data. After pulling up the training, DynamicProfilerMonitor will generate the configuration file profiler_config.json under the specified cfg_path, and the user can dynamically edit the configuration file, such as modifying it to the following configuration, which means that DynamicProfilerMonitor will start collecting performance data at the 10th step of training, and the online parsing will stop at the 10th step.
{ "start_step": 10, "stop_step": 10, "aicore_metrics": -1, "profiler_level": -1, "profile_framework": -1, "analyse_mode": 0, "profile_communication": false, "parallel_strategy": true, "with_stack": true, "data_simplification": false }
Example 2:DynamicProfilerMonitor is used in MindFormers.
Step 1: Add DynamicProfilerMonitor to MindFormers to register it to the training process. Modify the _build_profile_cb function in mindformers/trainer/trainer.py to change its default ProfileMonitor to DynamicProfilerMonitor, and the modification example is as follows.
def _build_profile_cb(self): """build profile callback from config.""" if self.config.profile: sink_size = self.config.runner_config.sink_size sink_mode = self.config.runner_config.sink_mode if sink_mode: if self.config.profile_start_step % sink_size != 0: self.config.profile_start_step -= self.config.profile_start_step % sink_size self.config.profile_start_step = max(self.config.profile_start_step, sink_size) logger.warning("profile_start_step should divided by sink_size, \ set profile_start_step to %s", self.config.profile_start_step) if self.config.profile_stop_step % sink_size != 0: self.config.profile_stop_step += self.config.profile_stop_step % sink_size self.config.profile_stop_step = max(self.config.profile_stop_step, \ self.config.profile_start_step + sink_size) logger.warning("profile_stop_step should divided by sink_size, \ set profile_stop_step to %s", self.config.profile_stop_step) start_profile = self.config.init_start_profile profile_communication = self.config.profile_communication # Add DynamicProfilerMonitor to replace the existing ProfileMonitor. from mindspore.profiler import DynamicProfilerMonitor # The cfg_path parameter is the path to the folder where the configuration file is to be shared, which needs to be accessible by all nodes in a cluster scenario # The output_path parameter is the path where the dynamic profile data is saved. profile_cb = DynamicProfilerMonitor(cfg_path="./dyn_cfg", output_path="./dynprof_data") # The original ProfileMonitor is no longer used. # profile_cb = ProfileMonitor( # start_step=self.config.profile_start_step, # stop_step=self.config.profile_stop_step, # start_profile=start_profile, # profile_communication=profile_communication, # profile_memory=self.config.profile_memory, # output_path=self.config.profile_output, # config=self.config) logger.warning( "Please reduce the data sample size with 'num_samples' in MindSpore data format according to " "https://www.mindspore.cn/mindinsight/docs/zh-CN/master/performance_profiling_ascend.html.") logger.warning("In profiler mode, auto-tune will be turned off.") self.config.auto_tune = False self.config.profile_cb = profile_cb
Step 2: After enabling the profile function in the model's yaml configuration file and pulling up the training, DynamicProfilerMonitor will generate the configuration file profiler_config.json under the specified cfg_path path after pulling up the training, and the user can dynamically edit the configuration file, for example, modify it to the following configuration, which means that DynamicProfilerMonitor will start to collect at the 10th step of training and stop collecting at the 10th step to parse online.
{ "start_step": 10, "stop_step": 10, "aicore_metrics": -1, "profiler_level": -1, "profile_framework": -1, "analyse_mode": 0, "profile_communication": false, "parallel_strategy": true, "with_stack": true, "data_simplification": false }
Launch MindSpore Insight
The MindSpore Insight launch command can refer to MindSpore Insight Commands.
Training Performance
Users can access the Training Performance by selecting a specific training from the training list, and click the performance profiling link.

Figure:Overall Performance
The above figure displays the overall performance of the training, including the overall data of Step Trace, Operator Performance, Data Preparation Performance and Timeline. The data shown in these components include:
Step Trace: It will divide the training steps into several stages and collect execution time for each stage. The overall performance page will show the step trace graph.
Operator Performance: It will collect the execution time of operators and operator types. The overall performance page will show the pie graph for different operator types.
Data Preparation Performance: It will analyse the performance of the data input stages. The overall performance page will show the number of steps that may be the bottleneck for these stages.
Timeline: It will collect execution time for stream tasks on the devices. The tasks will be shown on the time axis. The overall performance page will show the statistics for streams and tasks.
Users can click the detail link to see the details of each components. Besides, MindSpore Insight will try to analyse the performance data, the assistant on the left will show performance tuning suggestions for this training.
Step Trace Analysis
Note
Step trace analysis only supports single-graph and multi-subgraphs scenarios in Graph mode, and does not support scenarios such as pynative, heterogeneous. Multi-subgraphs scenarios, step trace analysis only display total time.

Figure:Step Trace Analysis
The above figure displays the Step Trace page. The Step Trace detail will show the start/finish time for each stage. By default, it shows the average time for all the steps. Users can also choose a specific step to see its step trace statistics.
The graphs at the bottom of the page show the execution time of Step Interval, Forward/Backward Propagation and Step Tail (The time between the end of Backward Propagation and the end of Parameter Update) changes according to different steps, it will help to decide whether we can optimize the performance of some stages. Here are more details:
Step Interval is the duration for reading data from data queues. If this part takes long time, it is advised to check the data preparation for further analysis.
Forward and Backward Propagation is the duration for executing the forward and backward operations on the network, which handle the main calculation work of a step. If this part takes long time, it is advised to check the statistics of operators or timeline for further analysis.
Step Tail is the duration for performing parameter aggregation and update operations in parallel training. If the operation takes long time, it is advised to check the statistics of communication operators and the status of parallelism.
In order to divide the stages, the Step Trace Component need to figure
out the forward propagation start operator and the backward propagation
end operator. MindSpore will automatically figure out the two operators
to reduce the profiler configuration work. The first operator after
get_next
will be selected as the forward start operator and the
operator before the last all reduce will be selected as the backward end
operator. However, Profiler do not guarantee that the automatically
selected operators will meet the user’s expectation in all cases.
Users can set the two operators manually as follows:
Set environment variable
PROFILING_FP_START
to configure the forward start operator, for example,export PROFILING_FP_START=fp32_vars/conv2d/BatchNorm
.Set environment variable
PROFILING_BP_END
to configure the backward end operator, for example,export PROFILING_BP_END=loss_scale/gradients/AddN_70
.
Operator Performance Analysis
The operator performance analysis component is used to display the execution time of the operators(AICORE/AICPU/HOSTCPU) during MindSpore run.
AICORE:AI Core operator is the main component of the computing core of Ascend AI processor, which is responsible for executing vector and tensor related computation intensive operators. TBE (Tensor Boost Engine) is an extended operator development tool based on TVM (Tensor Virtual Machine) framework. Users can use TBE to register AI Core operator information.
AICPU:AI CPU operator is a kind of CPU operator (including control operator, scalar, vector and other general-purpose calculations) that AI CPU is responsible for executing Hisilicon SOC in Ascend processor. The same operator in MindSpore may have AI Core operator and AI CPU operator at the same time. The framework will give priority to AI Core operator. If there is no AI Core operator or the selection is not satisfied, AI CPU operator will be called.
HOSTCPU:The host side CPU is mainly responsible for distributing the graph or operator to Ascend chip, and the operator can also be developed on the host side CPU according to the actual needs. The host CPU operator refers to the operator running on the host side CPU.

Figure:Statistics for Operator Types
The above figure displays the statistics for the operator types, including:
Choose pie or bar graph to show the proportion time occupied by each operator type. The time of one operator type is calculated by accumulating the execution time of operators belonging to this type.
Display top 20 operator types with the longest execution time, show the proportion and execution time (us) of each operator type.
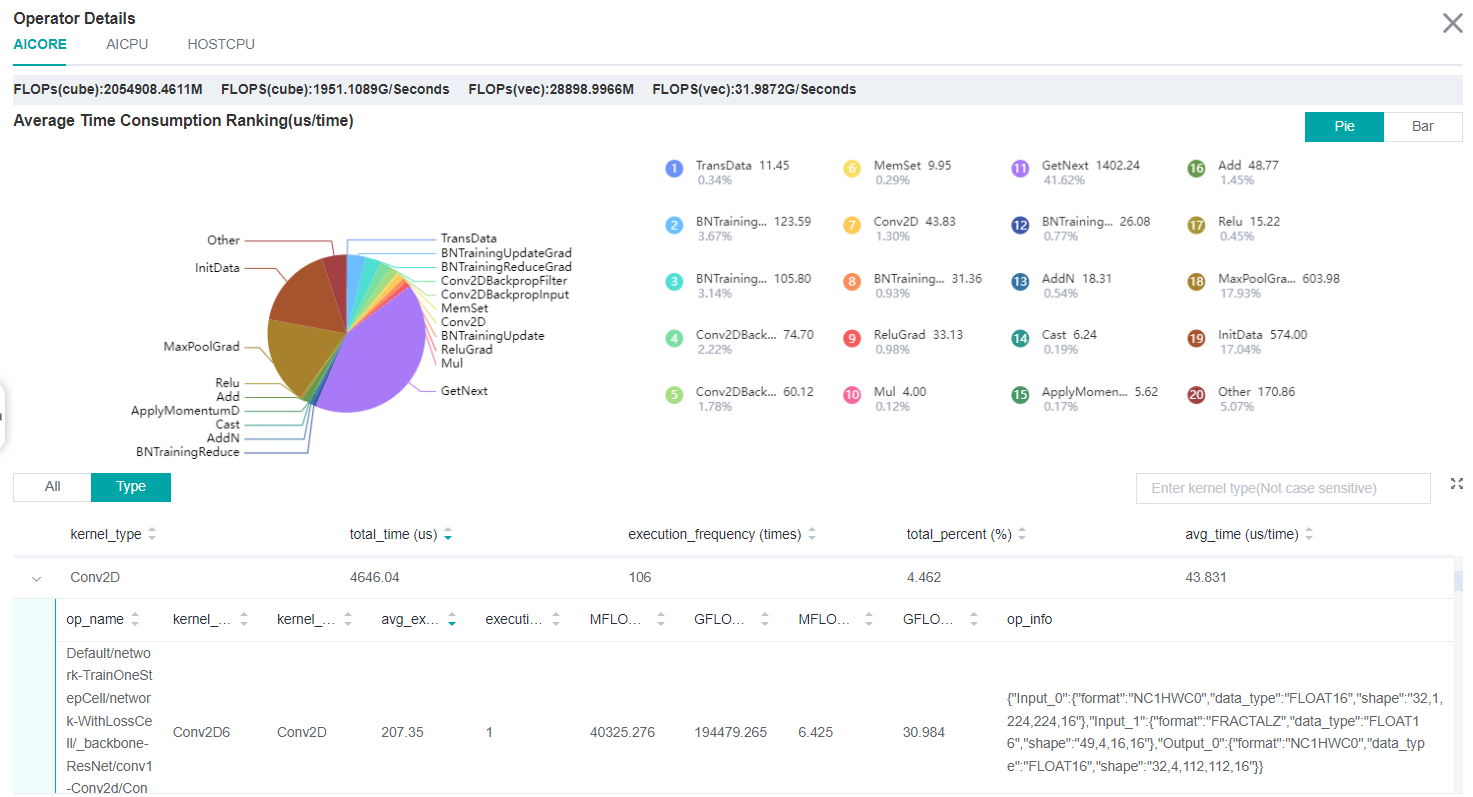
Figure:Statistics for Operators
The above figure displays the statistics table for the operators, including:
Choose All: Display statistics for the operators, including operator name, type, average execution time, execution frequency, full scope time, information, etc. The table will be sorted by execution time by default.
Choose Type: Display statistics for the operator types, including operator type name, execution time, execution frequency and proportion of total time. Users can click on each line, querying for all the operators belonging to this type.
Search: There is a search box on the right, which can support fuzzy search for operators/operator types.
Calculation quantity analysis
The Calculation Quantity Analysis module shows the actual calculation quantity data, including calculation quantity data for operator granularity and model granularity. The actual calculation quantity refers to the amount of calculation that is running on the device, which is different from the theoretical calculation quantity. For example, the matrix computing unit on the Atlas training series device is dealing with a matrix of 16x16 size, so in the runtime, the original matrix will be padded to 16x16. Only calculation quantity on AICORE devices is supported currently. The information about calculation quantity has four indicators:
FLOPs(cube): the number of cube floating point operations (the unit is million).
FLOPS(cube): the number of cube floating point operations per second (the unit is billion).
FLOPs(vec): the number of vector floating point operations (the unit is million).
FLOPS(vec): the number of vector floating point operations per second (the unit is billion).
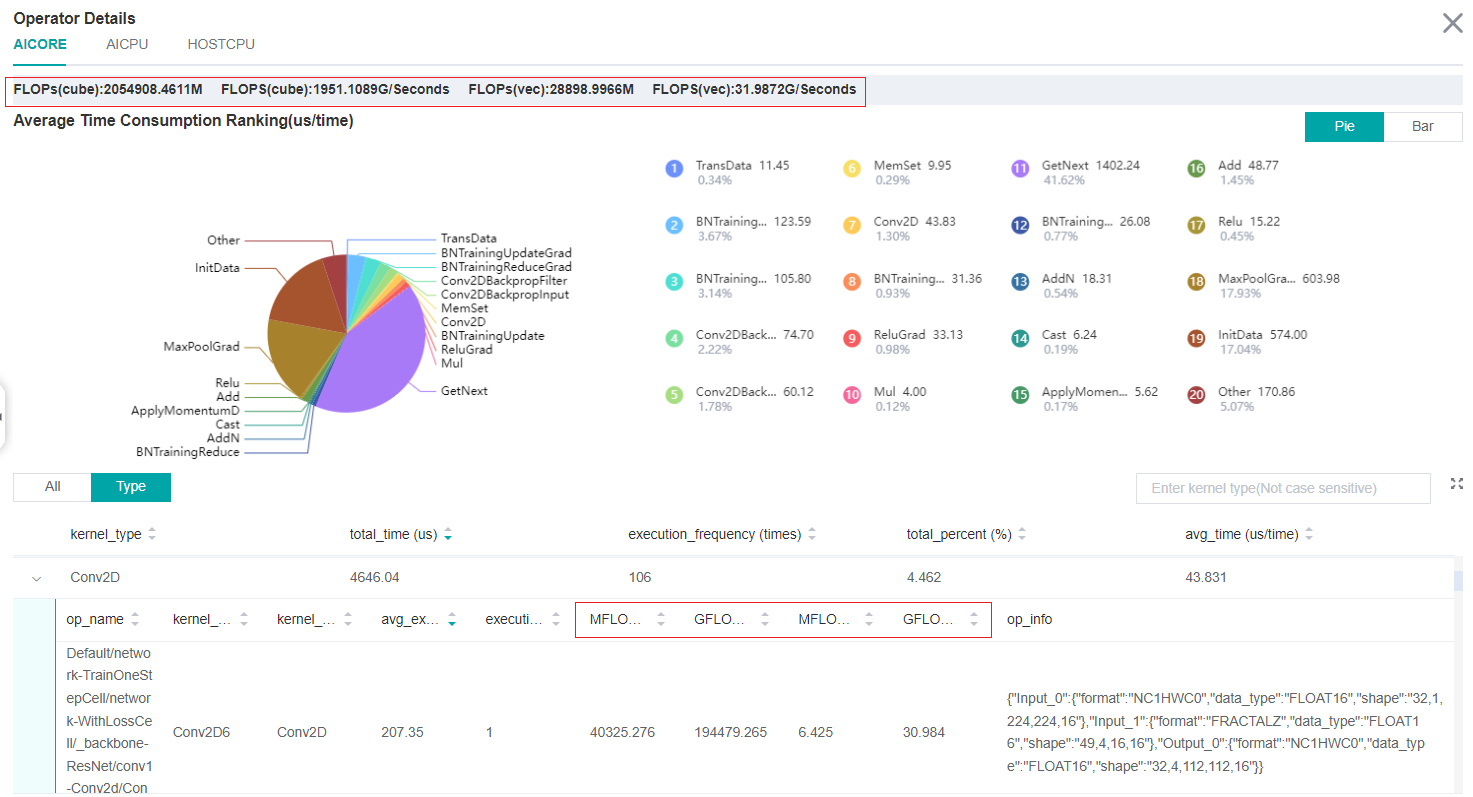
Figure:Calculation Quantity Analysis
The red box in figure above includes calculation quantity data on operator granularity and model granularity.
Data Preparation Performance Analysis

Figure:Data Preparation Performance Analysis
The above figure displays the page of data preparation performance analysis component. It consists of two tabs: the step gap and the data process.
The step gap page is used to analyse whether there is performance bottleneck in the three stages. We can get our conclusion from the data queue graphs:
The data queue size stands for the queue length when the training fetches data from the queue on the device. If the data queue size is 0, the training will wait until there is data in the queue; If the data queue size is greater than 0, the training can get data very quickly, and it means data preparation stage is not the bottleneck for this training step.
The host queue size can be used to infer the speed of data process and data transfer. If the host queue size is 0, it means we need to speed up the data process stage.
If the size of the host queue is always large and the size of the data queue is continuously small, there may be a performance bottleneck in data transfer.
Note
The queue size is the value recorded when fetching data, and obtaining the data of host queue and data queue is executed asynchronously, so the number of host queue steps, data queue steps, and user training steps may be different.
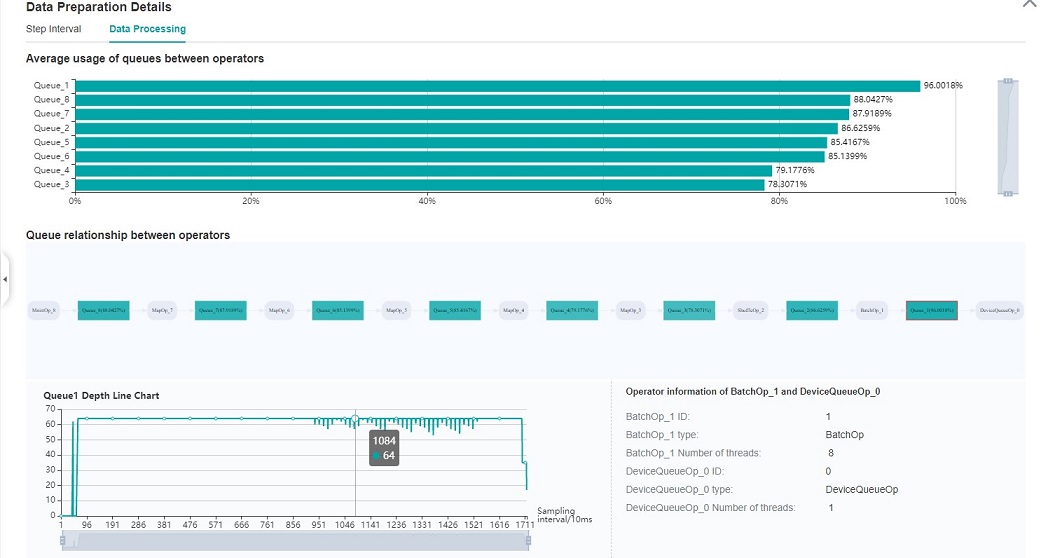
Figure:Data Process Pipeline Analysis
The above figure displays the page of data process pipeline analysis. The data queues are used to exchange data between the data processing operations. The data size of the queues reflect the data consume speed of the operations, and can be used to infer the bottleneck operation. The queue usage percentage stands for the average value of data size in queue divide data queue maximum size, the higher the usage percentage, the more data that is accumulated in the queue. The graph at the bottom of the page shows the data processing pipeline operations with the data queues, the user can click one queue to see how the data size changes according to the time, and the operations connected to the queue. The data process pipeline can be analysed as follows:
When the input queue usage percentage of one operation is high, and the output queue usage percentage is low, the operation may be the bottleneck.
For the leftmost operation, if the usage percentage of all the queues on the right are low, the operation may be the bottleneck.
For the rightmost operation, if the usage percentage of all the queues on the left are high, the operation may be the bottleneck.
To optimize the performance of data processing operations, there are some suggestions:
If the Dataset Loading Operation is the bottleneck, try to increase the
num_parallel_workers
.If the GeneratorOp Operation is the bottleneck, try to increase the
num_parallel_workers
or try to replace it withMindRecordDataset
.If the MapOp Operation is the bottleneck, try to increase the
num_parallel_workers
. If it maps a Python operation, try to optimize the training script.If the BatchOp Operation is the bottleneck, try to adjust the size of
prefetch_size
.
Note
To obtain data to prepare performance data, using the module of MindSpore Dataset to define data preprocessing pipeline.
Timeline Analysis
Timeline Features:
This feature is designed for the comparison and analysis of large model scenarios with multiple cards, iterations, and graphs.
Inspired by Nsight, it was first proposed to split data into two parts: summary and detail. The summary is positioned to showcase the overall execution of the model, while the detail is positioned to showcase the API level execution of the network.
The summary data include: step trace, overlap analysis of communication and computation; The detail data include: except for summary data, the execution order of calculation operators and communication operators.
Support filtering and merging data based on card number (rank id).
Support filtering and merging data based on multiple graphs (graph id).
How to view the timeline:
Click on the download button in the Timeline section of the overview page, download the timeline data(json format) locally.
Figure:Timeline download page
As shown in the figure above:
ranks: used for filtering and merging, default to all.
subgraphs: used for filtering subgraphs, default to all.
data kind: choice summary or detail, default to summary.
merge multiple subgraphs: whether to merge the iteration trajectory data of multiple subgraphs.
Open perfetto website, drag the downloaded timeline data onto the page to complete the display.
Figure:Timeline(2 ranks)Analysis
As shown in the figure above:
Step Trace: Display the forward and backward time and iteration trailing time of each iteration according to the dimension of graph and iteration.
Overlap Analysis: Including total network computing time, communication time, communication time not covered by computation, and card idle time.
Ascend Hardware: Display the execution order of device side calculation operators and communication operators according to the stream.
HCCL: Display the execution order of communication operators according to the plane.
Recommended usage of perfetto:
W/A/S/D can be applied to zoom in and out of the Timeline graph.
Select any event block, can view the detailed information of this block in the pop-up details bar below.
Mouse over multiple event blocks, can compare and analyze the execution time of multiple event blocks in the pop-up details bar below.
How to use timeline to solve practical problems:
Firstly, we recommend filtering and download summary data containing all ranks and graphs, identify performance bottlenecks based on overall network execution to avoid premature optimization.
Then, by filtering and downloading detailed data for certain ranks and graphs, further identify performance bottlenecks at the API level and identify optimization points.
After optimizing the code, repeat the step 1 and 2 above until the performance meets the requirements.
Dynamic Shape Iteration Analysis
When the training network is a dynamic shape network, the execution time of each operator (including AICPU operator and AICORE operator) during the operation of MindSpore can be statistically displayed by using the operator time-consuming (by iteration) component. It can quickly understand the time fluctuation of each operator in each iteration of training and the shape information of the operator in different iterations.
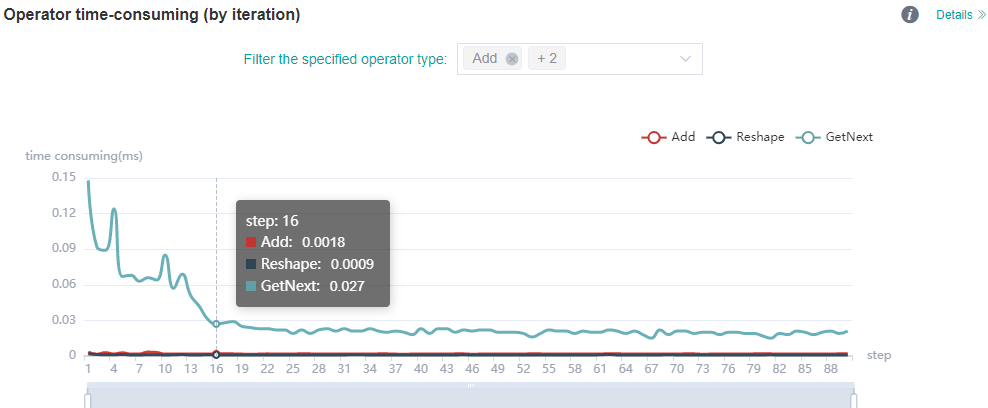
Figure: statistics of operator time (by iteration)
The figure above shows the analysis details of iteration time of different types of operators. You can view the iteration time curve of the specified operator type by filtering the specified operator type (the time shown here is the average time of the execution of different operator types).
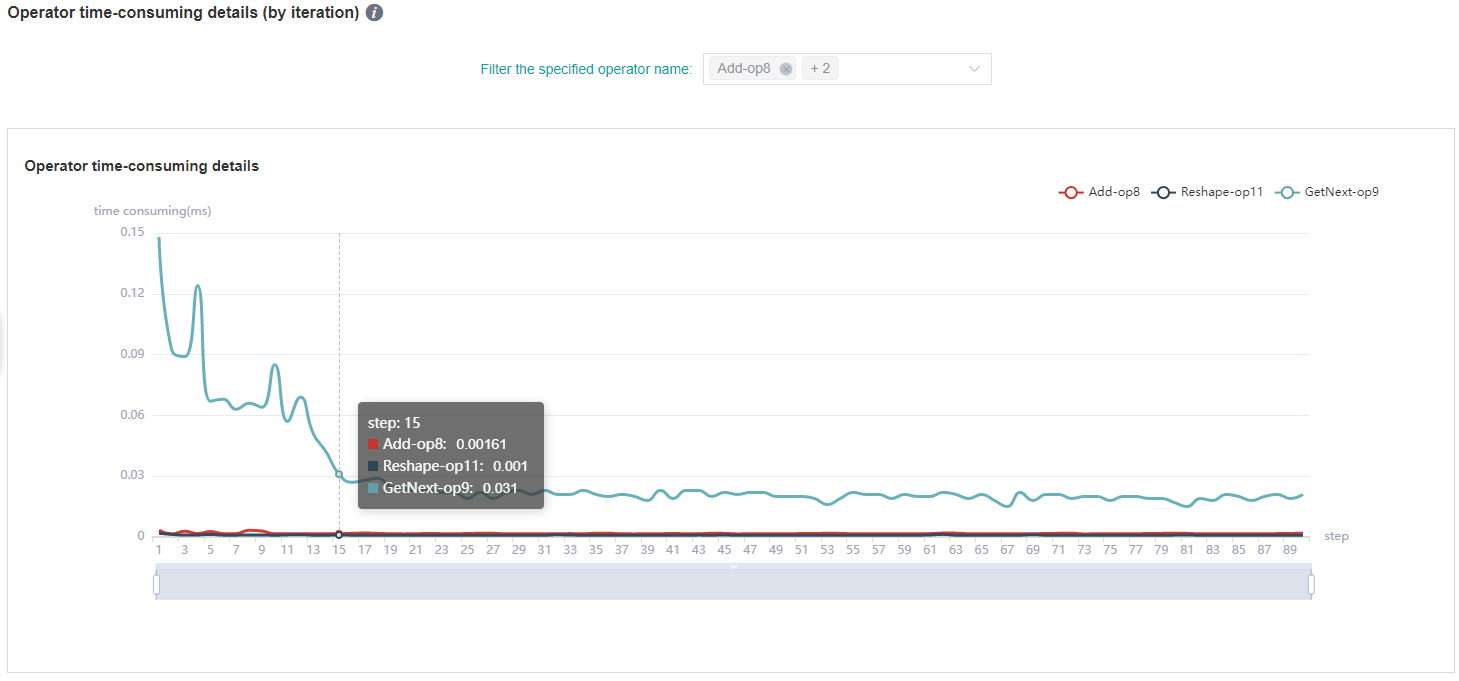
Figure: statistics of operator time-consuming details (by iteration)
The figure above shows the analysis details of iteration time of different operator instances. By filtering the specified operator name, the iteration time curve of the specified operator instance can be viewed.
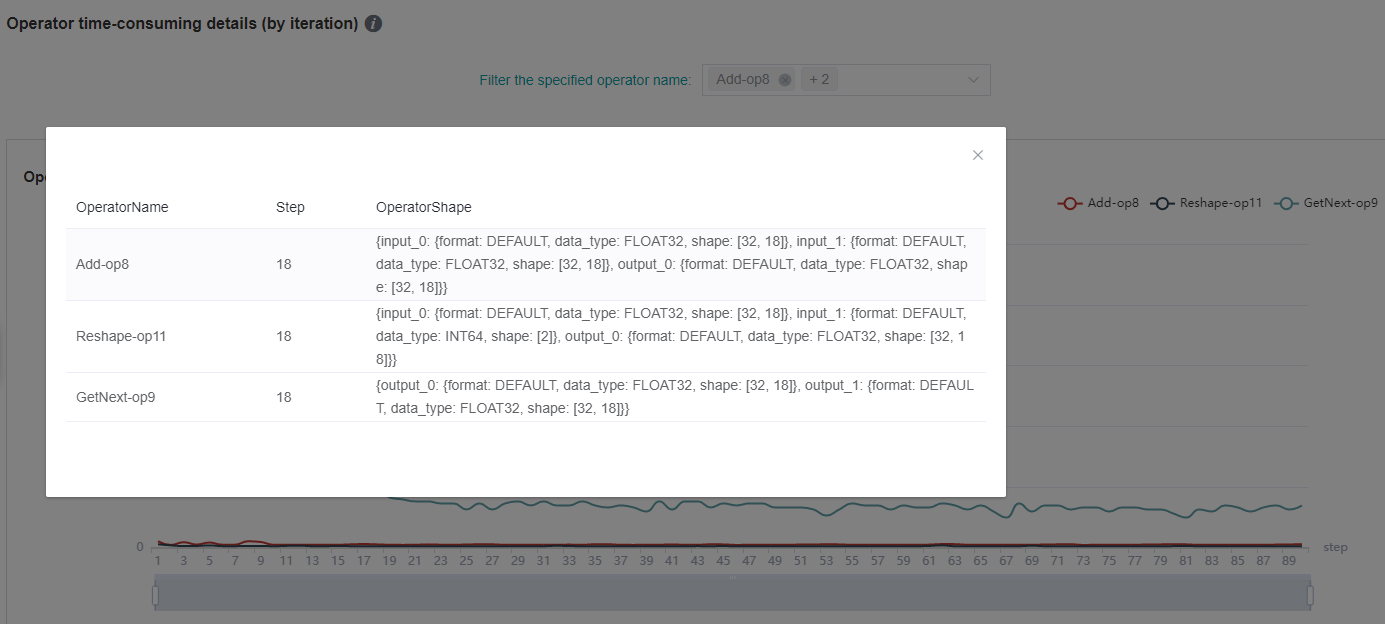
Figure: Shape information of operator (by iteration)
The figure above shows the shape information of the operator of a specific step. Click the corresponding point of the curve to check the shape information of the specified operator instance.
Note
Dynamic Shape network currently only supports the function modules of operator time (by iteration), operator time statistics ranking, data preparation, timeline, CPU utilization and parallel strategy, but does not support the functions of step trace, memory usage and cluster communication.
Msprof Tool to Assist Analysis
Users can collect detailed data of AI Core and On-Chip Memory/DDR read write rate data and PCIe bandwidth data through profiler, and then analyze and view them through Msprof tool.
The sample code is as follows:
profiler = Profiler(output_path='./data', aicore_metrics=1, l2_cache=True, hbm_ddr=True, pcie=True)
aicore_metrics is used to set the AI Core metric type, l2_cache is used to set whether to collect l2 cache data, hbm_ddr is used to set whether to collect On-Chip Memory/DDR read and write rate data, and pcie is used to set whether to collect PCIe bandwidth data. For parameter details, please refer to the API documentation.
MindSpore Profiler supports the collection of network performance data through the Msprof command line. For details about how to use the Msprof tool to collect and parse network performance data, please refer to the Msprof Collect General Commands
chapter of the CANN Development Tools Guide document.
Host Side Time Consumption Analysis
If the Host side time collection function is enabled, you can view the time consumption in ascend_timeline_display_[rank_id].json after the traing finished and use `` chrome://tracing `` to display. You can use W/S/A/D to zoom in, out, move left, and right to view time consuming information.
Resource Utilization
Resource utilization includes cpu usage analysis and memory usage analysis.
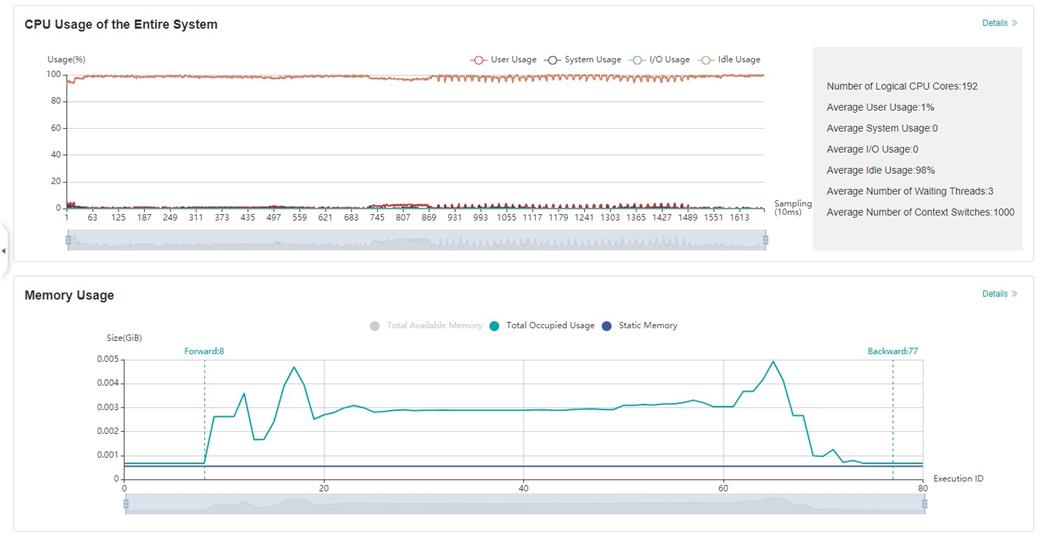
Figure:Overview of resource utilization
Overview of resource utilization:Including CPU utilization analysis and
memory usage analysis. You can view the details by clicking the View
Details
button in the upper right corner.
CPU Utilization Analysis
CPU utilization, which is mainly used to assist performance debugging. After the performance bottleneck is determined according to the queue size, the performance can be debugged according to the CPU utilization (if the user utilization is too low, increase the number of threads; if the system utilization is too high, decrease the number of threads). CPU utilization includes CPU utilization of the whole machine, process and Data pipeline operation.
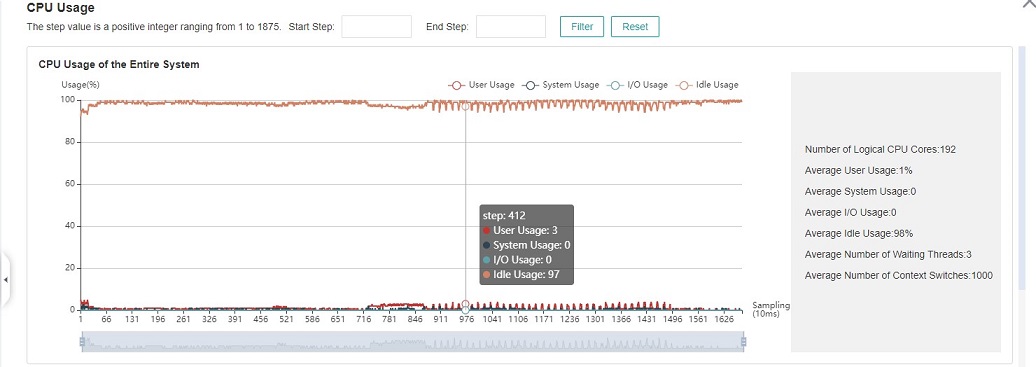
Figure:CPU utilization of the whole machine
CPU utilization of the whole machine: Show the overall CPU usage of the device in the training process, including user utilization, system utilization, idle utilization, IO utilization, current number of active processes, and context switching times. If the user utilization is low, you can try to increase the number of operation threads to increase the CPU utilization; if the system utilization is high, and the number of context switching and CPU waiting for processing is large, it indicates that the number of threads needs to be reduced accordingly.
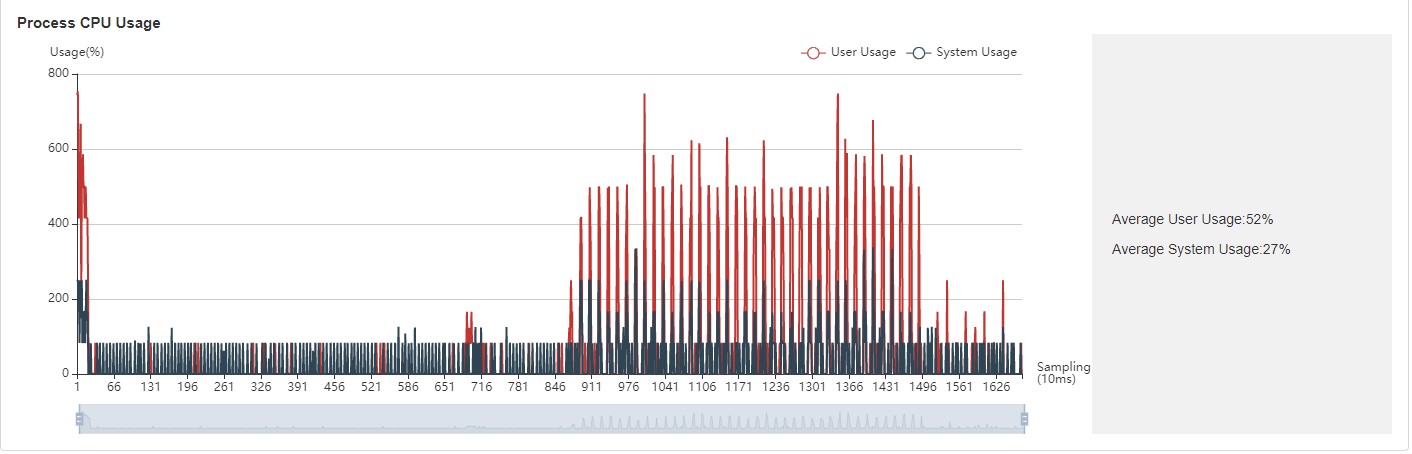
Figure:Process utilization
Process utilization: Show the CPU usage of a single process. The combination of whole machine utilization and process utilization can determine whether other processes affect the training process.
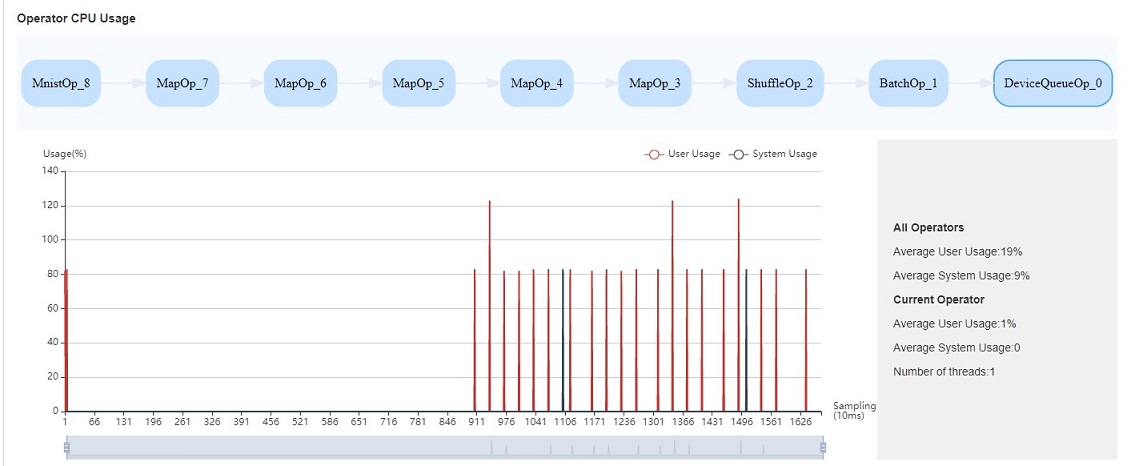
Figure:Operator utilization
Operator utilization: Show the CPU utilization of Data pipeline single operation. We can adjust the number of threads of the corresponding operation according to the actual situation. If the number of threads is small and takes up a lot of CPU, you can consider whether you need to optimize the code.
Common scenarios of CPU utilization:
According to the queue size, the network debugging personnel can judge that the performance of MindData has a bottleneck. They can adjust the number of threads by combining the utilization rate of the whole machine and the utilization rate of the operator.
Developers can check the utilization of operators. If an operation consumes CPU utilization, they can confirm whether the code needs to be optimized.
Note
The default sampling interval is 1000ms. You can change the sampling
interval through
mindspore.dataset.config.get_monitor_sampling_interval()
. For
details:
Memory Analysis
This page is used to show the memory usage of the neural network model on the device, which is an ideal prediction based on the theoretical calculation results. The content of the page includes:
An overview of the memory usage of the model, including the total available memory, peak memory and other information.
The memory occupied varies in the execution order while the model is running.
The memory usage of each operator is decomposed and displayed in the table of
Operator Memory Allocation
.
Note
Memory Analysis does not support heterogeneous training currently.
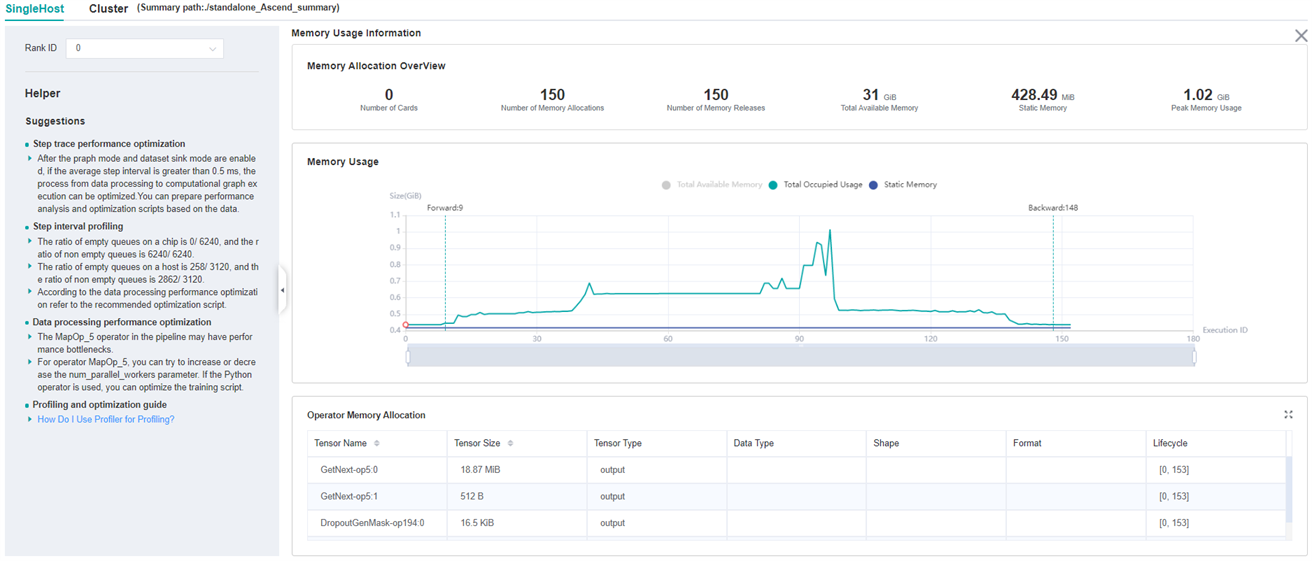
Figure:Memory Analysis
Users can obtain the summary of memory usage via the
Memory Allocation Overview
. In addition, they can obtain more
detailed information from Memory Usage
, including:
Zooming: There is a zoom scroll bar under the line chart. Users can zoom in or out the line chart by adjusting its size to observe more details.
FP/BP: The execution positions of the start of
Forward Propagation
and the end ofBackward Propagation
of the model on the line chart.Details of Nodes: Hovering over the line chart, the information of the corresponding execution operator is shown, including the execution order of the operator, the name of the operator, the memory occupied by the operator, the total memory occupied by the model in the current position, and the relative memory change compared with the previous execution position.
Memory Decomposition: Left clicking a position on the line chart, the memory breakdowns of the execution position is shown in the table below the line chart, called
Operator Memory Allocation
. The table shows the memory decomposition of the corresponding execution position, i.e., the output tensor of which operators are allocated the occupied memory of the current execution position. The module provides users with abundant information, including tensor name, tensor size, tensor type, data type, shape, format, and the active lifetime of tensor memory.
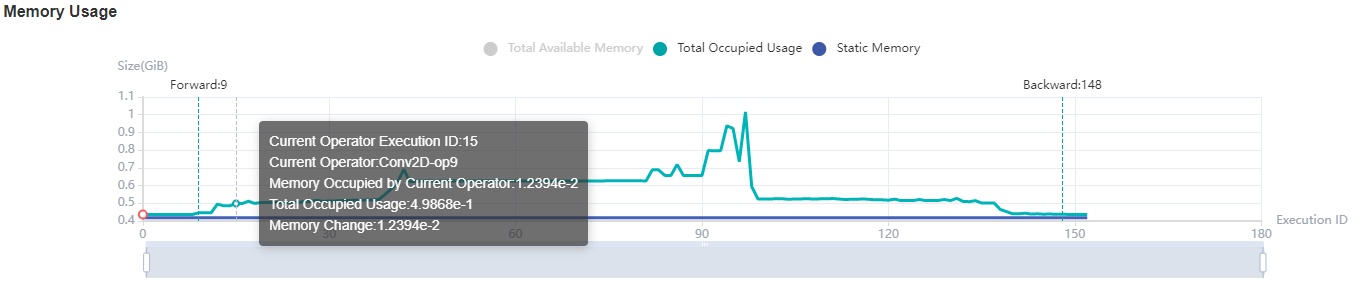
Figure:Memory Statistics
Offline Analyse
When the Profiler collects a large volume of performance data, directly utilizing Profiler.analyse() for online parsing during the training process may result in excessive system resource usage, thereby impacting training efficiency. The Profiler offers an offline parsing capability, allowing for the use of Profiler.offline_analyse to parse collected data after the profiling is complete.
A code example of the training script that collects performance data without online parsing is as follows:
class Net(nn.Cell):
...
def train(net):
...
if __name__ == '__main__':
ms.set_context(mode=ms.GRAPH_MODE, device_target="Ascend")
# Init Profiler
# Note that the Profiler should be initialized before model.train
profiler = ms.Profiler(output_path='/path/to/profiler_data')
# Train Model
net = Net()
train(net) # Error occur.
# Collection end
profiler.stop()
Following the collection of performance data in the aforementioned code, the data can be parsed using an offline parsing interface. Here is an example of the code:
from mindspore import Profiler
Profiler.offline_analyse(path='/path/to/profiler_data', pretty=False, step_list=None, data_simplification=True)
The offline parsing interface parameter descriptions are as follows:
path (str): The profiling data path which need to be analyzed offline. There needs to be a profiler directory in this path.
pretty (bool, optional): Whether to pretty json files. Default:
False
.step_list (list, optional): A list of steps that need to be analyzed. Default:
None
. By default, all steps will be analyzed.data_simplification (bool, optional): Whether to enable data simplification. Default:
True
.
Notes on parameters:
The step_list parameter is only effective when parsing data collected in graph mode, and the specified steps must be consecutive, with the step range starting from 1 based on the actual number of collected steps. For example: if 5 steps are collected, the available range is [1,2,3,4,5].
The data_simplification parameter is enabled by default. If this switch is turned on for two consecutive offline parsings, the first data simplification will delete the framework-side collected data, resulting in the absence of framework-side parsing results in the second offline parsing.
The offline parsing of the input path supports both single-card and multi-card data paths, with different scenarios described as follows.
Single-card scenario
When using offline parsing to analyze single-card data, the directory structure of the profiling data path /path/to/profiler_data is as follows:
└──── profiler_data
└────profiler
The parsed performance data is generated under the /path/to/profiler_data/profiler directory.
Multi-card scenario
When using offline parsing to analyze multi-card data, the directory structure of the profiling data path /path/to/profiler_data is as follows:
└──── profiler_data
├────rank_0
│ └────profiler
├────rank_1
│ └────profiler
├────rank_2
│ └────profiler
└────rank_3
└────profiler
The parsed performance data is generated under the profiler directory of each card's data path. The following shows the directory structure of the generated performance data.
Directory Structure
An example of the performance data catalog structure is shown below:
└──── profiler
├──── container
├──── FRAMEWORK // Raw data collected on the frame side
│ └──── op_range_*
├──── PROF_{number}_{timestamp}_{string} // msprof performance data
│ ├──── analyse
│ ├──── device_*
│ ├──── host
│ ├──── mindstudio_profiler_log
│ └──── mindstudio_profiler_output
├──── rank_* // Memory-related raw data
│ ├──── memory_block.csv
│ └──── task.csv
├──── rank-*_{timestamp}_ascend_ms // MindStudio Insight Visualization Deliverables
│ ├──── ASCEND_PROFILER_OUTPUT // Performance data collected by the MindSpore Profiler interface
│ ├──── profiler_info_*.json
│ └──── profiler_metadata.json // To record user-defined meta data, call the add_metadata or add_metadata_json interface to generate the file
├──── aicore_intermediate_*_detail.csv
├──── aicore_intermediate_*_type.csv
├──── aicpu_intermediate_*.csv
├──── ascend_cluster_analyse_model-{mode}_{stage_num}_{rank_size}_*.csv
├──── ascend_timeline_display_*.json
├──── ascend_timeline_summary_*.json
├──── cpu_framework_*.txt // Heterogeneous scenario generation
├──── cpu_ms_memory_record_*.txt
├──── cpu_op_detail_info_*.csv // Heterogeneous scenario generation
├──── cpu_op_execute_timestamp_*.txt // Heterogeneous scenario generation
├──── cpu_op_type_info_*.csv // Heterogeneous scenario generation
├──── dataset_iterator_profiling_*.txt // Data non-sinking scenario generation
├──── device_queue_profiling_*.txt // Data sinking scenario generation
├──── dynamic_shape_info_*.json
├──── flops_*.txt
├──── flops_summary_*.json
├──── framework_raw_*.csv
├──── hccl_raw_*.csv // Configure the profiler (profiler_communication=True) to generate
├──── minddata_aicpu_*.json // Data sinking scenario generation
├──── minddata_cpu_utilization_*.json
├──── minddata_pipeline_raw_*.csv
├──── minddata_pipeline_summary_*.csv
├──── minddata_pipeline_summary_*.json
├──── operator_memory_*.csv
├──── output_timeline_data_*.txt
├──── parallel_strategy_*.json
├──── pipeline_profiling_*.json
├──── profiler_info_*.json
├──── step_trace_point_info_*.json
└──── step_trace_raw_*_detail_time.csv
└──── dataset_*.csv
* represents rank id
Performance Data File Description
PROF_{number}_{timestamp}_{string} directory is the performance data collected by CANN Profiling, which is mainly stored in mindstudio_profiler_output. The data introduction can be found in the Ascend Community Official Website by searching for "Profile Data File Overview".
The profiler directory contains three types of files, csv, json, and txt, which cover performance data in terms of operator execution time, memory usage, communication, etc. The file descriptions are shown in the following table. For detailed descriptions of some files, refer to Performance data.
File Names |
Descriptions |
---|---|
step_trace_point_info_*.json |
Information about the operator corresponding to the step node (only mode=GRAPH,export GRAPH_OP_RUM=0) |
step_trace_raw_*_detail_time.csv |
Time information for the nodes of each STEP (only mode=GRAPH,export GRAPH_OP_RUM=0) |
dynamic_shape_info_*.json |
Operator information under dynamic shape |
pipeline_profiling_*.json |
MindSpore data processing to capture intermediate files of falling disks for MindInsight visualization |
minddata_pipeline_raw_*.csv |
MindSpore data processing to capture intermediate files of falling disks for MindInsight visualization |
minddata_pipeline_summary_*.csv |
MindSpore data processing to capture intermediate files of falling disks for MindInsight visualization |
minddata_pipeline_summary_*.json |
MindSpore data processing to capture intermediate files of falling disks for MindInsight visualization |
framework_raw_*.csv |
Information about AI Core operators in MindSpore data processing |
device_queue_profiling_*.txt |
MindSpore data processing to capture intermediate files of falling disks for MindInsight visualization (data sinking scenarios only) |
minddata_aicpu_*.txt |
Performance data for AI CPU operators in MindSpore data processing (data sinking scenarios only) |
dataset_iterator_profiling_*.txt |
MindSpore data processing to capture intermediate files of falling disks for MindInsight visualization (data non-sinking scenarios only) |
aicore_intermediate_*_detail.csv |
AI Core operator data |
aicore_intermediate_*_type.csv |
AI Core operator calling counts and time taken statistics |
aicpu_intermediate_*.csv |
Time taken data after AI CPU operator information parsing |
flops_*.txt |
Record the number of floating-point calculations (FLOPs), floating-point calculations per second (FLOPS) for AI Core operators |
flops_summary_*.json |
Record total FLOPs for all operators, average FLOPs for all operators, average FLOPS_Utilization |
ascend_timeline_display_*.json |
timeline visualization file for MindStudio Insight visualization |
ascend_timeline_summary_*.json |
timeline statistics |
output_timeline_data_*.txt |
Operator timeline data, only if AI Core operator data exists |
cpu_ms_memory_record_*.txt |
Raw files for memory profiling |
operator_memory_*.csv |
Operator-level memory information |
minddata_cpu_utilization_*.json |
CPU utilization rate |
cpu_op_detail_info_*.csv |
CPU operator time taken data (mode=GRAPH only) |
cpu_op_type_info_*.csv |
Class-specific CPU operator time taken statistics (mode=GRAPH only) |
cpu_op_execute_timestamp_*.txt |
CPU operator execution start time and time taken (mode=GRAPH only) |
cpu_framework_*.txt |
CPU operator time taken in heterogeneous scenarios (mode=GRAPH only) |
ascend_cluster_analyse_model-xxx.csv |
Data related to computation and communication, etc. in model-parallel or pipeline-parallel modes (mode=GRAPH only) |
hccl_raw_*.csv |
Card-based communication time and communication wait time (mode=GRAPH only) |
parallel_strategy_*.json |
Operator parallel strategy to capture falling disk intermediate files for MindInsight visualization |
profiler_info_*.json |
Profiler Configuration and other info |
dataset_*.csv |
The time consuming of various stages of data processing module (To collect these must turn the profiler on from the beginning of the task at least before the step 1 starts) |
* represents rank id
The complete name of ascend_cluster_analyse_model-xxx_*.csv should be ascend_cluster_analyse_model-{mode}_{stage_num}_{rank_size}_{rank_id}.csv, such as ascend_cluster_analyse_model-parallel_1_8_0.csv
Specifications
To limit the data size generated by the Profiler, MindSpore Insight suggests that for large neural network, the profiled steps should be less than 10.
Note
The number of steps can be controlled by controlling the size of training dataset. For example, the
num_samples
parameter inmindspore.dataset.MindDataset
can control the size of the dataset. For details, please refer to: dataset API .The parse of Timeline data is time consuming, and usually the data of a few steps is enough to analyze the results. In order to speed up the data parse and UI display, Profiler will show at most 20M data (Contain 10+ step information for large networks).
Enabling the profiler has a partial performance impact on the training process. If the impact is significant, data collection items can be reduced. The following is a comparison of the performance of the Resnet network before and after enabling the profiler:
network:Resnet
Disable profiler
Enable profiler
Performance Comparison
1P+PYNATIVE
31.18444ms
31.67689ms
+0.49245ms
1P+GRAPH
30.38978ms
31.72489ms
+1.33511ms
8P+PYNATIVE
30.046ms
32.38599ms
+2.33999ms
8P+GRAPH
24.06355ms
25.04324ms
+0.97969ms
The performance data in the chart shows the average time spent on one step during the training process of the resnet network on Atlas training series. (Note: There are performance fluctuations in network training, and the above data is for reference only)
Notices
Currently the training and inference process does not support performance debugging, only individual training or inference is supported.
Step trace analysis only supports single-graph and multi-subgraphs scenarios in Graph mode, and does not support scenarios such as pynative, heterogeneous.
Multi-subgraphs scenarios, step trace analysis only display total time.
Enable profiling based on step, enable profiling based on epoch, step trace analysis and cluster analysis are only supported in Graph mode.
MindSpore Profiler Python API cannot enable profiling with the
PROFILING_MODE
environment variable.