# TensorRT Integration Information [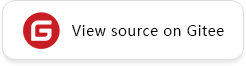](https://gitee.com/mindspore/docs/blob/r1.8/docs/lite/docs/source_en/use/tensorrt_info.md) ## Steps ### Environment Preparation Besides basic [Environment Preparation](https://www.mindspore.cn/lite/docs/en/r1.8/use/build.html), CUDA and TensorRT is required as well. Current version only supports CUDA version 10.1 and TensorRT version 6.0.1.5. Install[CUDA 10.1](https://developer.nvidia.com/cuda-10.1-download-archive-base), set the installed directory to environment viriable as `${CUDA_HOME}`. Our build script uses this environment viriable to seek CUDA. Install[TensorRT 6.0.1.5](https://developer.nvidia.com/nvidia-tensorrt-6x-download), set the installed directory to environment viriable as `${TENSORRT_PATH}`. Our build script uses this environment viriable to seek TensorRT. ### Build In the Linux environment, use the build.sh script in the root directory of MindSpore [Source Code](https://gitee.com/mindspore/mindspore) to build the MindSpore Lite package integrated with TensorRT. First configure the environment variable `MSLITE_GPU_BACKEND=tensorrt`, and then execute the compilation command as follows. ```bash bash build.sh -I x86_64 ``` For more information about compilation, see [Linux Environment Compilation](https://www.mindspore.cn/lite/docs/en/r1.8/use/build.html#linux-environment-compilation). ### Integration - Integration instructions When developers need to integrate the use of TensorRT features, it is important to note: - [Configure the TensorRT backend](https://www.mindspore.cn/lite/docs/en/r1.8/use/runtime_cpp.html#configuring-the-gpu-backend), For more information about using Runtime to perform inference, see [Using Runtime to Perform Inference (C++)](https://www.mindspore.cn/lite/docs/en/r1.8/use/runtime_cpp.html). - Compile and execute the binary. If you use dynamic linking, please refer to [Compilation Output](https://www.mindspore.cn/lite/docs/en/r1.8/use/build.html#directory-structure) with compilation option `-I x86_64`. Please set environment variables to dynamically link related libs. ```bash export LD_LIBRARY_PATH=mindspore-lite-{version}-{os}-{arch}/runtime/lib/:$LD_LIBRARY_PATH export LD_LIBRARY_PATH=user-installed-tensorrt-path/lib/:$LD_LIBRARY_PATH export LD_LIBRARY_PATH=user-installed-cuda-path/lib/:$LD_LIBRARY_PATH ``` - Using Benchmark testing TensorRT inference Pass the build package to a device with a TensorRT environment(TensorRT 6.0.1.5) and use the Benchmark tool to test TensorRT inference. Examples are as follows: - Test performance ```bash ./benchmark --device=GPU --modelFile=./models/test_benchmark.ms --timeProfiling=true ``` - Test precision ```bash ./benchmark --device=GPU --modelFile=./models/test_benchmark.ms --inDataFile=./input/test_benchmark.bin --inputShapes=1,32,32,1 --accuracyThreshold=3 --benchmarkDataFile=./output/test_benchmark.out ``` For more information about the use of Benchmark, see [Benchmark Use](https://www.mindspore.cn/lite/docs/en/r1.8/use/benchmark.html). For environment variable settings, you need to set the directory where the `libmindspore-lite.so` (under the directory `mindspore-lite-{version}-{os}-{arch}/runtime/lib`), TensorRT and CUDA `so` libraries are located, to `${LD_LIBRARY_PATH}`. - Using TensorRT engine serialization TensorRT backend inference supports serializing the built TensorRT model (Engine) into a binary file and saves it locally. When it is used the next time, the model can be deserialized and loaded from the local, avoiding rebuilding and reducing overhead. To support this function, users need to use the [LoadConfig](https://www.mindspore.cn/lite/api/en/r1.8/generate/classmindspore_Model.html) interface to load the configuration file in the code, you need to specify the saving path of serialization file in the configuration file: ``` [ms_cache] serialize_path=/path/to/config ``` ## Supported Operators For supported TensorRT operators, see [Lite Operator List](https://www.mindspore.cn/lite/docs/en/r1.8/operator_list_lite.html).